This article was reviewed by Joe Cursons, PhD, from immuno-oncology startup oNKo-innate.
Stay up to date on the latest science with Brush Up Summaries.
What Is Multiomics?
Multiomics is a multidisciplinary, holistic approach to biological analysis in which scientists integrate and analyze different types of omics data from a single sample.1 The omes commonly featured in multiomics analysis include the genome, epigenome, transcriptome, proteome, metabolome, and microbiome.2
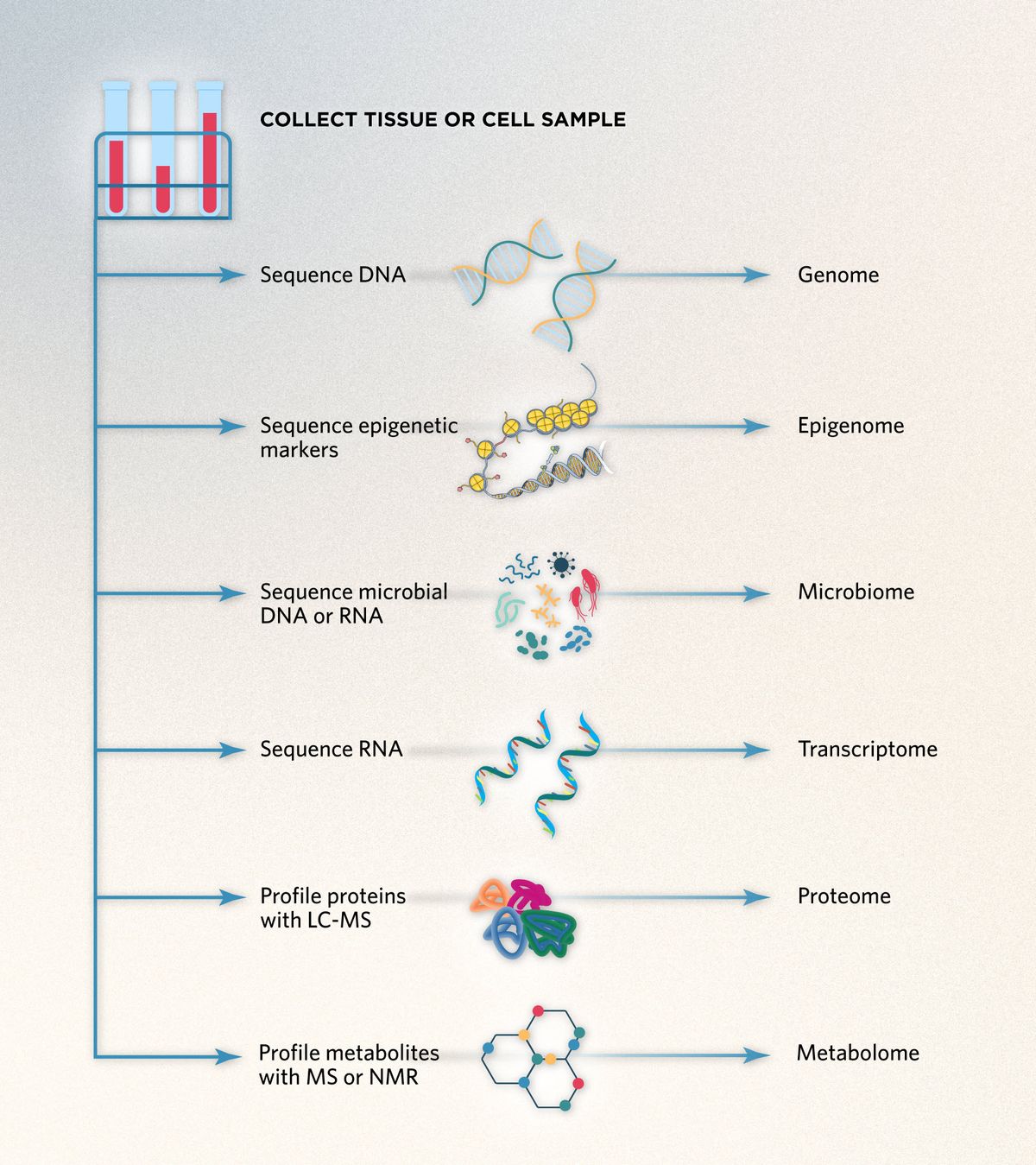
Each of these standalone omics technologies offers detailed insight into a particular group of molecules, such as DNA, RNA, or proteins, but has a restricted view of the entire biological system.1 By combining two or more of these large-scale omics datasets, scientists can form a comprehensive understanding of the biological complexity of the sample and explore relationships between the different omes.
“The collection and comparison of different types of omics data allows us to look at, for example, whether there are any interactions between changes in the transcription of [a gene] and the changes in the abundance of the corresponding protein, or whether changes in histone modifications at a certain site in the DNA correspond to changes in the transcription of that target gene,” explained Joe Cursons, a computational biologist at immuno-oncology startup oNKo-innate, who uses multiomics to understand the immune response to solid tumors.
Approaches to Multiomics
Approaches to multiomics analysis differ considerably based on which omes are included and whether the samples are individual cells or larger tissues. Recent advances in sequencing methods have allowed scientists to study multiomics at the single-cell level,3 analyzing minute quantities of various molecules. In contrast, spatial multiomics enables them to study the different omes in the context of their native tissues.4
For example, a researcher might perform a multiomics workflow to compare the genome, epigenome, transcriptome, and proteome of a muscle tissue sample from a patient with muscular dystrophy. To do so, they could split a muscle tissue biopsy into four smaller samples and perform the following experiments to generate four different omics datasets.
- Sample 1 – Genome: Extract DNA and perform whole-genome sequencing
- Sample 2 – Epigenome: Extract DNA for bisulfite sequencing of DNA methylation patterns and nanopore sequencing of histone modifications
- Sample 3 – Transcriptome: Extract RNA to execute RNA sequencing
- Sample 4 – Proteome: Isolate proteins and subject them to liquid chromatography-mass spectrometry (LC-MS) analysis
Several commercial sequencing methods used in multiomics research can perform more than one type of omics analysis simultaneously, including ATAC-seq, which simultaneously profiles the transcriptome and epigenome,5 and CITE-seq, which sequences the transcriptome and proteome.6 “A big contributor to the increase in multiomics recently has been the growing availability of these commercial kits,” said Cursons. Scientists also use 16S rRNA gene sequencing to study the diversity of microbes that live symbiotically or pathogenically in human tissues, making up the microbiome.2
Multiomics Analysis
Researchers perform data integration through bioinformatics and computational biology pipelines, analyzing different omics datasets generated by high-throughput technologies. Multiomics researchers typically analyze data independently using programs such as Python or R to avoid the shortcomings of summary statistics from individual omics pipelines.
“A critical consideration with multiomics is that you really need to plot the data [yourself],” Cursons explained. “Quite often, the different measurement technologies that you're using for these different types of omics will have very different sources of noise, and the best statistical models for that noise might vary between different types of data.” Because of the complexity, heterogeneity, and scale of multiomics data, machine learning tools are becoming increasingly common in multiomics analysis.7
In the example of muscular dystrophy, this integrated multiomics approach allows researchers to explore the molecular continuum from gene to protein, revealing complex mechanisms and pathways that underpin the muscular dystrophy phenotype and identifying potential targets for treatment.
Targets identified in multiomics analyses typically require further experiments to validate them or confirm causality, such as gene knockouts. “It's sometimes quite hard with multiomics methods to necessarily identify the cause and effect,” remarked Cursons. “What's the chicken, and what's the egg? Is it the changes in the transcription causing the changes in the histone markers? Or is it the changes in the histone markers changing the gene expression? That sometimes gets a bit blurry unless you go in and do a perturbation experiment.”
For example, a researcher could silence a specific target at the genetic level to confirm it as a driver of disease. If a gene knockout or similar perturbation does not yield downstream changes as seen in a multiomics analysis, the researcher can investigate the other identified targets as potential drivers.
Multiomics Applications
Scientists apply multiomics across a variety of research areas, using it to solve real-world problems.
Multiomics in agriculture and aquaculture
Agricultural researchers have used multiomics to understand mechanisms of growth, senescence, yield, and stress responses in plants, identifying targets for crop improvement in a range of valuable species.8
In aquaculture, scientists have applied integrated transcriptomic and metabolomic analyses to identify the molecular mechanism underlying carp muscle quality changes when they are farmed in different aquaculture systems.9 In a study of farmed salmon treated for infection using chemotherapeutic agents, scientists used multiomics to reveal pathways involved in the physiological adaptation of the fish to treatment-induced oxidative stress.10
Multiomics in biomedicine
Advances in multiomics technology have caused a revolution in medical science, identifying complex pathogenesis causes, disease diagnostic biomarkers, and therapeutic targets.1 “[Scientists] often talk about someone's DNA or their genome being their blueprint, but it's the interaction of a person's genome together with their environment that ultimately controls the outcome or the emergence of disease,” said Cursons.
Cancer researchers like Cursons often use a multiomics approach to unravel the regulatory pathways involved in tumorigenesis or the immune response to cancer. For example, researchers have used genome, transcriptome, and proteome data to identify subtypes of kidney cancer.2 Multiomics approaches have also been used to identify biomarkers for many cancers that could not be identified using stand-alone omics approaches, and to predict survival in liver cancer.2
By including the microbiome in their multiomics analysis, researchers can elucidate previously unknown disease pathogenesis and treatment resistance mechanisms in cancer, or identify microbiome-based biomarkers that can predict treatment response.11 “There's now a massive amount of evidence that the composition of the microbes in a person's gut can significantly influence how they respond to [immune checkpoint blockade drugs such as] PD-L1 and PD-1 inhibitors, which are increasingly used in cancer immunotherapy,” Cursons explained.
References
1. Gutierrez Reyes CD, et al. Multiomics applications in biological systems. Curr Issues Mol Biol. 2024;46(6):5777-5793.
2. Chen C, et al. Applications of multi-omics analysis in human diseases. MedComm. 2023;4(4):e315.
3. Chappell L, et al. Single-cell (multi)omics technologies. Annu Rev Genomics Hum Genet. 2018;19:15-41.
4. Vandereyken K, et al. Methods and applications for single-cell and spatial multi-omics. Nat Rev Genet. 2023;24(8):494-515.
5. Grandi FC, et al. Chromatin accessibility profiling by ATAC-seq. Nat Protoc. 2022;17(6):1518-1552.
6. Lakkis J, et al. A multi-use deep learning method for CITE-seq and single-cell RNA-seq data integration with cell surface protein prediction and imputation. Nat Mach Intell. 2022;4(11):940-952.
7. Nicora G, et al. Integrated multiomics analyses in oncology: a review of machine learning methods and tools. Front Oncol. 2020;10.
8. Yang Y, et al. Applications of multiomics technologies for crop improvement. Front Plant Sci. 2021;12.
9. Wang L, et al. Multiomics reveals the molecular mechanism of muscle quality changes in common carp (Cyprinus carpio) under two aquaculture systems. Comp Biochem Physiol Part D Genomics Proteomics. 2024;52:101290.
10. Lazado CC, et al. Multiomics provide insights into the key molecules and pathways involved in the physiological adaptation of atlantic salmon (Salmo salar) to chemotherapeutic-induced oxidative stress. Antioxidants (Basel, Switzerland). 2021;10(12).
11. Gunjur A, et al. A gut microbial signature for combination immune checkpoint blockade across cancer types. Nat Med. 2024;30(3):797-809.
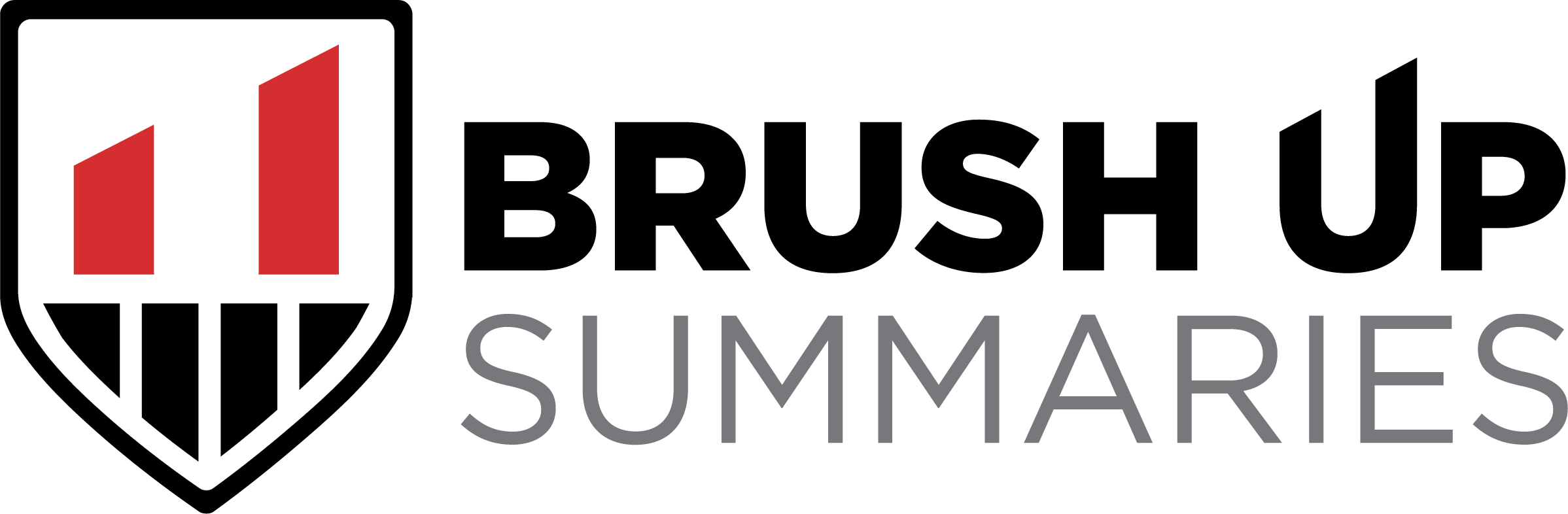