above: © istock.com, jurgenfr
“Research parasite.” When a pair of physicians publicized the term in a 2016 editorial in the New England Journal of Medicine, it was an inflammatory way to call out researchers who didn’t generate their own data but instead used data produced by others to make novel discoveries. Increasing data sharing, the authors warned, would lead to a parasite proliferation.
But not everyone agreed with the perspective. Casey Greene, a genomics researcher at the University of Pennsylvania, read the editorial and thought “it was essentially describing a good scientist—someone who looks skeptically at other people’s data,” he says. “I thought it was an opportunity to take the absurd, which was the sort of idea that those people are parasites, and turn it into a more productive conversation, which is the idea that this is actually a good practice and should be recognized.”
Thus were born...
Some research suggests that simply publishing in journals with open-data policies improves statistical rigor and reproducibility because authors know their findings will be exposed to scrutiny.
Lighthearted awards aside, some research organizations have advocated for greater sharing of raw data from published studies. Funders such as the Bill and Melinda Gates Foundation and the Chan Zuckerberg Initiative have explicit open-data requirements for any research they support, and journals such as Science, Nature, and many more also now have open-data policies.
Although there is a gathering consensus that data openness is a boon to scientific progress, there remains disagreement within the scientific community about how and when to share. Some argue that the researchers who invested time, dollars, and effort in producing data should have exclusive rights to analyze the data and publish their findings. Others point out that data sharing is difficult to enforce in any case, leading to an imbalance in who benefits from the practice—a problem that some researchers say has yet to be satisfactorily resolved.
Open-data policies boost scientific research
No biological field has seen a faster rise in, or greater benefit from, data sharing than genomics. In the early 2000s, when micro-array technology appeared, and researchers could suddenly measure the activity of tens of thousands of genes at once, fear swept through the scientific community that false positives would become unacceptably common and pollute the literature with spurious findings. To ensure that results were genuine, the research community and journals publishing this type of data quickly came to realize that sharing was a condition of credibility, Greene explains. That led to a culture of openness that continues today, with specially built data repositories, such as GenBank, the Sequence Read Archive, and the database of Genotypes and Phenotypes (dbGaP), designed to collate and share genomic data.
One major advantage of these databases has been their cost effectiveness in the long run. “We estimate that maybe there’s something in the neighborhood of three million publicly available genome-wide assays at the moment,” Greene says. “If you consider conservatively that each one costs at least one thousand bucks to make, that’s about three billion dollars of data.” Rather than individual researchers having to bear part of that cost every time they conduct a study, “if the data are public, then anyone with a computer and internet connection can test their ideas on the dataset,” says microbiome researcher Florian Fricke of the University of Hohenheim in Stuttgart, Germany.
Shared access to genetic data also allows individual researchers to pursue projects that might not have immediate appeal to funding agencies, notes Julie Dunning Hotopp, a genome scientist at the University of Maryland. She won the 2018 Sustained Parasitism award from Greene’s award committee for studies using existing genomic data-sets to conclusively demonstrate that bacterial DNA integrates into insect chromosomes—a controversial idea before she published the findings. “People don’t want to fund things that might not work,” Hotopp tells The Scientist. Reusing other people’s data allows researchers “to ask questions we couldn’t get funding for because they’re just too expensive, or they’re controversial.”
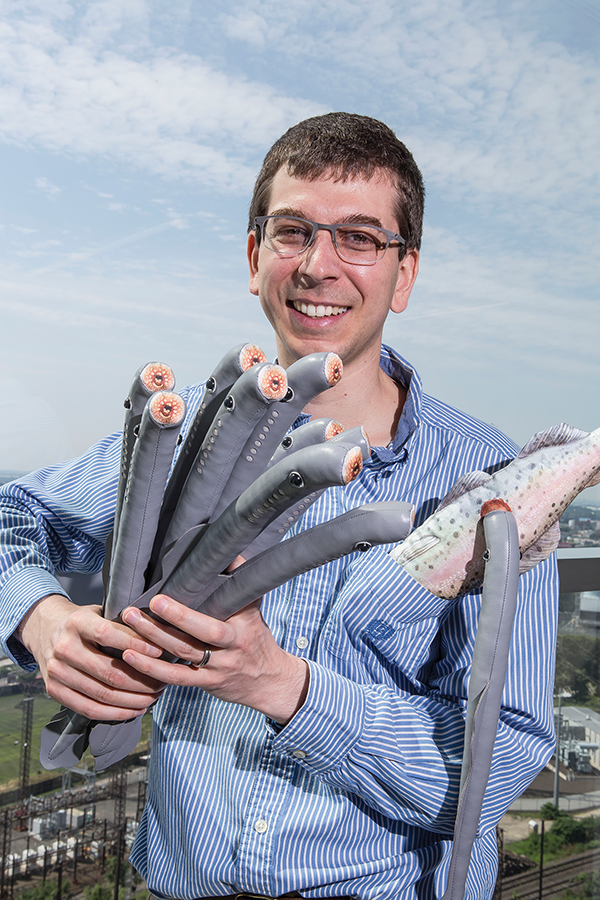
With greater sharing comes greater volumes of data, too. Large datasets have been particularly valuable in clinical research, where meta-analyses combining raw data from several trials prove far more informative than the findings of any individual study. Analyzing multiple datasets simultaneously might give a researcher the statistical power to study a treatment effect in subcategories of patients, or to identify subtle effects that are only detectable in larger sample sizes, for example.
Of course, data sharing also allows researchers to check each other’s work, a critical part of verifying experiment-al findings. The Center for Open Science,
for instance, is conducting several large-scale projects to assess reproducibility in psychology, cancer biology, and other fields. The Science Exchange also has a reproducibility initiative to independently validate key experimental results in biological sciences, particularly in cancer bio-logy, where lab findings may help launch expensive clinical trials.
Even when scientists don’t set out to scrutinize data that have been made publicly available by other groups, some studies suggest that the mere act of data sharing may itself raise research standards, says John Ioannidis, professor of medicine and of health research and policy at Stanford University. In a recent study published in the BMJ, Ioannidis and his coauthors reanalyzed clinical trials published in the BMJ and PLOS Medicine, journals that require authors to share raw data as a prerequisite to publication. Although the team detected some errors in the reanalyses, on the whole those errors were minor—they didn’t change the conclusions of the papers.
That’s in contrast to the findings of a study Ioannidis published in JAMA in 2014. He and his colleagues reviewed published reanalyses of clinical trial data—mostly from trials that had not made data freely available at the time of publication. Almost all of the 37 reanalyses were carried out by teams that included at least one of the authors from the original study, but more than a third led to an interpretation that differed from that of the original publication. For example, “the original paper would say the drug is effective, and the reanalysis would say the drug is not effective, or it would say that it is effective for a completely different group of patients compared to the one that the original had suggested,” Ioannidis explains.
These findings hint that simply publishing in journals with open-data policies improves statistical rigor and reproducibility because authors know their findings will be exposed to scrutiny, he adds—a hypothesis supported by a 2011 study that found researchers’ reluctance to share data for a particular paper was associated with weaker statistical support and a higher prevalence of errors in reporting results.
Weighing the costs of data sharing
Even with all its advantages, data sharing is not without challenges. “One of the bigger problems with data sharing is it imposes a cost on the sharer, and gives them essentially no benefit,” says Greene.
Some of that cost is in the effort researchers have to expend making the data available. “There’s always some amount of extra hassle involved in preparing the data, and submitting it, and providing all the necessary metadata, and so on,” explains Anne Carpenter, an imaging scientist at the Broad Institute of Harvard and MIT whose lab is known for developing open-source image analysis software. “It’s a sacrifice for each lab to go through that effort.” Some researchers therefore may opt to make their data “available upon request” instead—a path that can lead to months of delays for other research groups trying to get a hold of what they need, Fricke says.
Even when researchers are willing to put in the time, it’s not always obvious what data format should be used. For Andre Brown, who studies the genomics of behavior using the nematode C. elegans as a model system at Imperial College London, the challenge is that his lab’s data usually consist of huge video files. There wasn’t any standard for how to analyze or share data like his, nor was there a public repository. To address this issue, Brown and his colleagues created an open-source platform for analyzing and sharing worm behavior data, and developed a new data format that is easier to share. He and other worm researchers, aided by citizen scientists, also created a searchable database to help C. elegans researchers easily identify the data they are most interested in. “Building the format and database took several years, but now that the infrastructure is in place, sharing subsequent datasets will take relatively little work,” he tells The Scientist.
It really is just about
changing expectations.—Anne Carpenter, Broad Institute
Then there’s the financial cost of maintaining shared databases. In 2011, for example, the National Institutes of Health (NIH) almost phased out the Sequence Read Archive, a public database of biological sequence data, because of budgetary constraints. Data storage in itself isn’t all that expensive; the majority of the cost comes from having to pay highly trained staff to maintain and curate the databases so that data can be easily searched, accessed, and used by others. Unless these costs decrease tremendously, there will have to be some sort of reckoning as to what data can be stored, notes Hotopp. “I don’t think we can store every piece of data we’ve ever generated,” she says.
There are also concerns regarding sharing of human research subjects’ data. Clinical trial data are de-identified and the risk of re-identifying study participants is low, says Ioannidis, so sharing shouldn’t pose a privacy risk. But a 2015 study suggests that when research participants consider the implications of open-data policies, they are concerned about confidentiality, anonymity, and data security. Such policies may also make it difficult to enforce the terms of informed consent: if research participants’ data is published in one of the increasingly numerous journals with open-data policies, they could potentially be reused for studies to which participants did not consent.
Finally, of course, there’s what some researchers perceive as the risk of being scooped. Last July, neuroscientist Jack Gallant became embroiled in a Twitter spat about why he hadn’t released the data from a 2016 Nature study of how the meanings of words are represented in the human brain. His students and postdocs at the University of California, Berkeley, were still analyzing the data for further studies, he wrote; the data would be shared “very soon.” Some researchers responded in the Twitter thread to argue that that wasn’t a sufficient reason, and that the data should be made publicly available right away; others have argued elsewhere that forcing researchers to share their data before they are done with their analyses amounts to data “leeching and thievery.”
Moving open-data policy forward
Despite disagreements about when and how to share, the trend toward data openness shows no signs of abating. Funders will have an important role in catalyzing progress, Greene says. For instance, grant agencies such as the NIH currently do not factor in data-sharing plans when evaluating the impact of a grant application. “It’s all about the finding, and nothing about the resources—whether those are data or new protocols,” Greene says. He is one of several researchers who argue that more funders should instead incentivize data-sharing practices by including sharing plans in impact scores for competitive proposals.
The NIH “has a longstanding commitment to making the results and accomplishments of the research that it funds and conducts available to the public,” David Kosub, a spokesperson for the NIH Office of Extramural Research, writes in an email to The Scientist. “Going forward, NIH is continuing to evaluate the most effective strategies to support and promote data sharing.”
The National Science Foundation, meanwhile, already considers Data Management Plans—in which grant applicants detail how they will disseminate their research data and metadata—to be “an integral part of all full proposals” that will be “considered under Intellectual Merit or Broader Impacts of both,” according to the agency’s Data Management Plan guidance.
Journals can also help turn data sharing into a standard part of the scientific publication process. For instance, in 2016, Cell Press introduced Structured, Transparent, Accessible Reporting (STAR) Methods—which require the sharing of all techniques, source data, and any other information needed to repeat an experiment—to improve the transparency and reproducibility of studies published in its journals. Fricke adds that the peer reviewers should check that data are available as part of the review process.
Ultimately, however, the incentive to move towards data openness is likely to come from within the research community itself. “It really is just about changing expectations for what people expect of you,” says Broad’s Carpenter. “It’s embarrassing if your lab is the only one that isn’t sharing data.”
Viviane Callier is a freelance science writer based in San Antonio, Texas.
Interested in reading more?
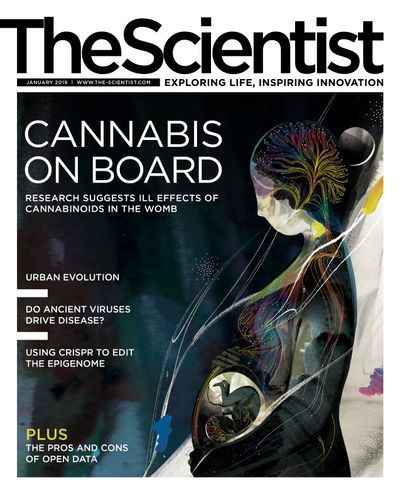