ABOVE: As the AI flood gates open, biology is experiencing a surge of new applications. From synthetic proteins to cell mapping, researchers are using AI to improve precision medicines. © istock.com, FRANKRAMSPOTT, BEST CONTENT PRODUCTION GROUP, MARINA DEKHNIK, JACKIE NIAM, NOBI_PRIZUE, PHONGSAK SANGKHAMANEE; DESIGNED BY ASHLEIGH CAMPSALL
In the early 1990s, protein biologists invested in solving a challenge that had riddled them for decades. The protein folding problem centered on the idea that biologists should be able to predict the three-dimensional structure of a protein based on its amino acid sequence, but they hadn’t been able to do so in practice. Researchers knew that the ability to determine protein structure without relying on tedious experiments would unlock a plethora of applications—better drug targets, easy protein function determination, and optimized industrial enzymes—so they persisted.
In 1994, a few researchers led by biophysicist John Moult from the University of Maryland started the biannual Critical Assessment of Protein Structure Prediction (CASP) competition as a large-scale experiment to source solutions from the collective. At every event, the brightest minds in protein biology brought forth their models that predicted structures of a few test proteins chosen by the organizers. The model that yielded structures that most closely resembled experimental data won.
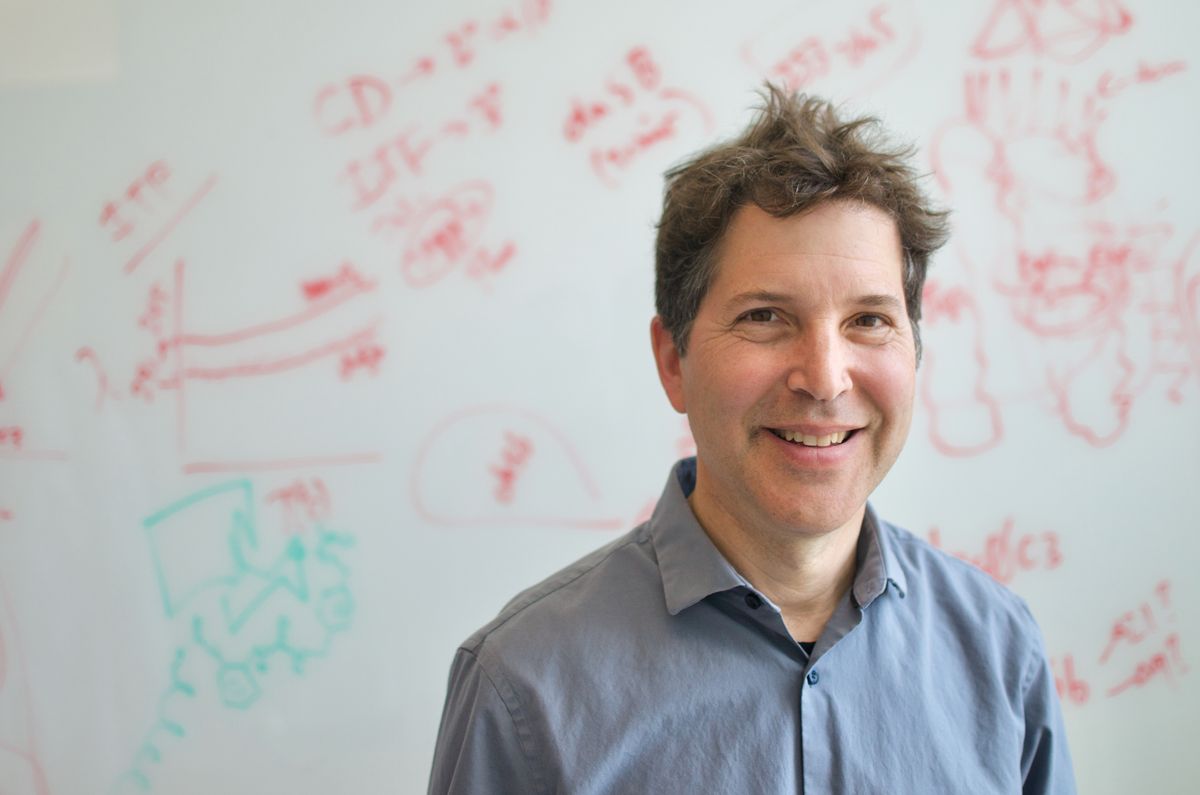
For the first several years, scientists relied on physical prediction models for these challenges, recalled David Baker, a protein design specialist at the University of Washington and a CASP competition contributor and advisor. “Proteins are made out of amino acid residues, which are made out of atoms, and you try and model all the interactions between the atoms and how they drive the protein to fold up,” Baker explained.
In 2018 at CASP13, the attendees witnessed a breakthrough. Demis Hassabis, cofounder and chief executive officer at DeepMind, an artificial intelligence company, and his team challenged the status quo by using a deep learning-based model to predict protein structure. They trained their model, AlphaFold, using the sequences and structures of about 100,000 known proteins to enable it to output pattern-recognition based predictions.1
AlphaFold won the competition that year, and the field progressed rapidly thereafter. By the next CASP meeting, the DeepMind team significantly improved their model, and AlphaFold predicted the structures of the majority of test proteins with an accuracy comparable to experimental methods.2 Based on AlphaFold’s success, protein experts declared that the 50-year old protein folding problem was largely solved. AlphaFold inspired researchers to pivot towards AI for their protein folding models; Baker and his team soon launched their open source deep learning-based protein structure predictor RoseTTA fold.3
While these models successfully predicted the structures of almost all existing proteins, Baker was interested in proteins beyond the database, including proteins that did not exist.
AI accelerates protein design
Baker has always been interested in tinkering with proteins and especially in designing new ones. “It wasn't too long after our first successes in structure prediction that we started thinking, well, maybe instead of predicting what structure a sequence would fold up to, we could use these methods to make a completely new structure and then find out what sequence could fold to it,” he said.
Why is it that Netflix is able to give you recommendations for what movies you're going to like to watch tonight, but your clinician can't get you AI guided recommendations for therapies for how you should be treated?
– Trey Ideker, University of California San Diego
He and his team developed their first de novo protein, an alpha/beta protein called Top7, using physical modeling methods in 2003.4 Over the years, Baker’s team and other researchers steadily expanded the list of de novo proteins.5 Now, with AI tools in their arsenal, researchers could design more complex proteins with a higher success rate, said Baker. Indeed, in the past few years, researchers, including Baker’s team, have reported different protein design models.6,7 The team involved in developing one of these models, ProGen, used it to design synthetic enzymes, lysozymes, as a proof of concept.8 Experimental tests revealed that the artificial lysozymes showed catalytic efficiencies matching natural ones, demonstrating the prowess of such models in building utilitarian proteins in the lab.
“The proteins in nature evolved under the constraints of natural selection. So, they solve all the problems that were relevant for natural selection during evolution. But now, we can make proteins specifically for 21st century problems. That is what is really exciting about the field,” said Baker.
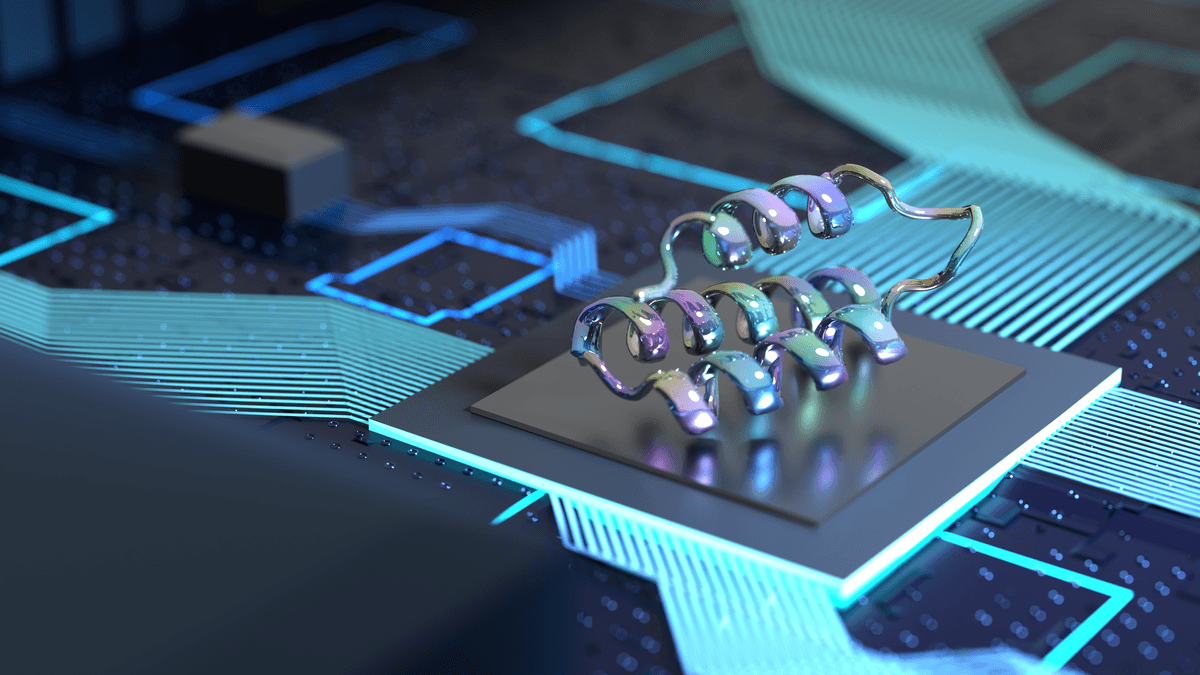
Baker’s team is tackling several such needs-of-the-hour projects. He recently developed a de novo coronavirus vaccine in collaboration with Neil King, who specializes in protein design at the University of Washington.9 His team also works on targeted cancer drugs, enzymes that break down plastic, and proteins to fix carbon dioxide.
There is always more work to be done. Proteins in cells are often part of macromolecular complexes. Current AI models work well for protein folding predictions or creating a protein with a specific binding site, but they fall short when it comes to designing more complicated complexes, such as molecular motors. “With the current methods, it's not so obvious how to design machines. That's still a research problem,” said Baker.
Building bridges: AI models map cells
According to Trey Ideker, a computational biologist and functional genomics researcher at the University of California, San Diego, the AI-driven progress in protein folding was a huge milestone for biologists. “That impact is still being felt,” he said. But it solved just a small part of a complex problem.
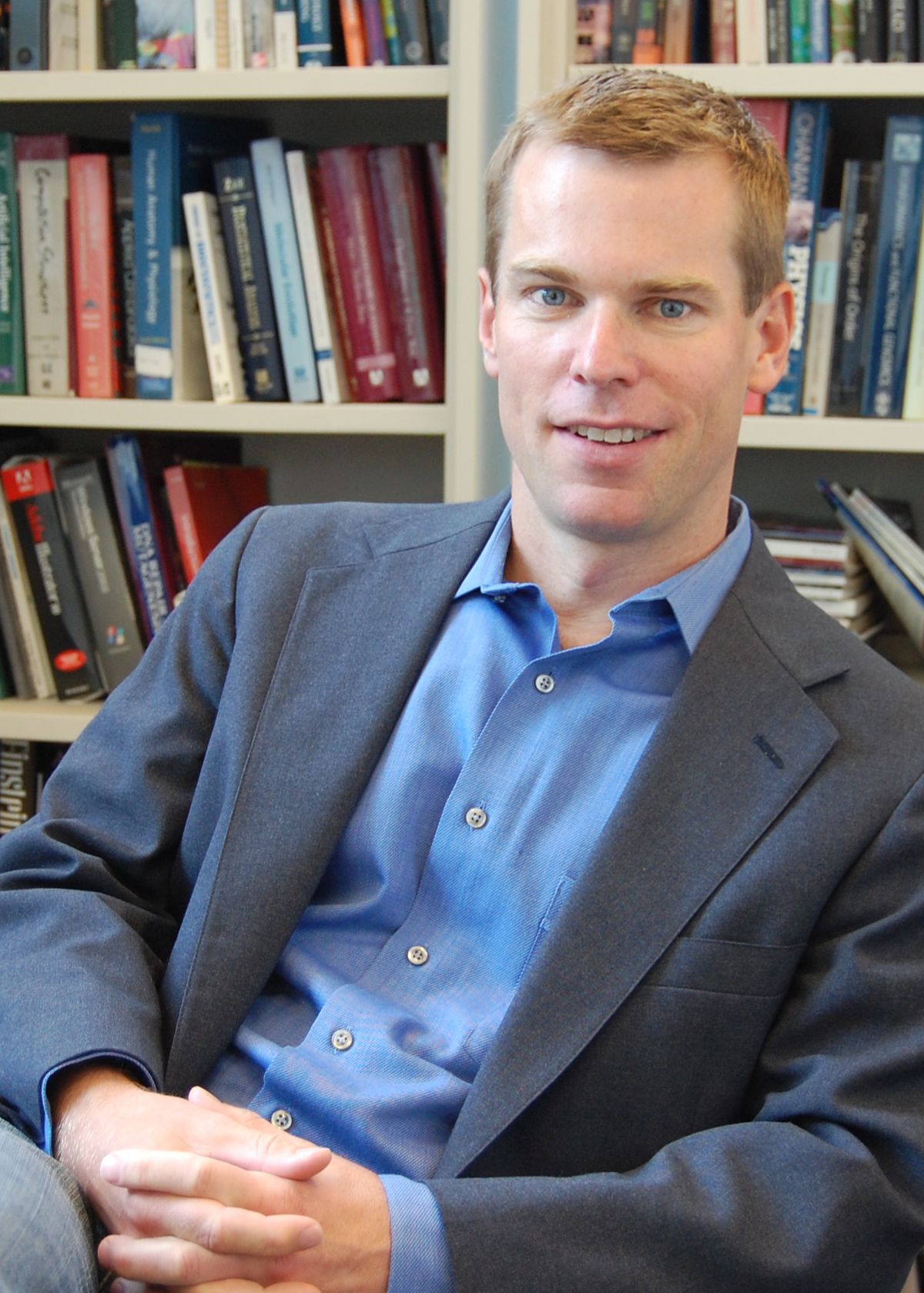
Proteins do not work alone; they interact with other proteins in intricate pathways to enable cellular function and structure. A deeper understanding of cell structure and its determinants will help researchers identify perturbations that indicate diseased states. While cell imaging provides a snapshot of cellular architecture, researchers are far from developing real cell maps and models, according to Ideker.
“How do you Alphafold a cell?” he questioned. “How would you fold an entire cell for every cell in your body?” Ideker intends to find the answers, and he has just the right resources to do so: a collaborative group of like-minded scientists.
As AI tools become more widespread in biology, many researchers have turned to deep learning models in their projects to improve precision medicine. With data at the crux of these models, it is vital to ensure that researchers have complete datasets to maximize their chances of success. With a goal of coordinating this progress, the NIH launched the Bridge2AI program with a focus on plugging in the key missing datasets that are needed to train future AI models to take them to the next level. “It's not AI yet; it's the bridge to AI,” said Ideker.
One focus project under this initiative is the Cell Maps for AI (CM4AI) program, which aims to build spatiotemporal maps of cells and connect genotype to phenotype to get a complete picture of cell health. The scientists involved in this program will achieve this by working on all aspects of cellular biology: genetic perturbations, cell imaging for morphology detection, and protein interaction studies. Ideker leads the functional genomics subgroup in the CM4AI program.
“I'm actually optimistic we're going to get there relatively soon. But a lot of work remains and needs continued innovations in AI and data measurements,” said Ideker.
Cellular image analysis: AI has an accurate eye
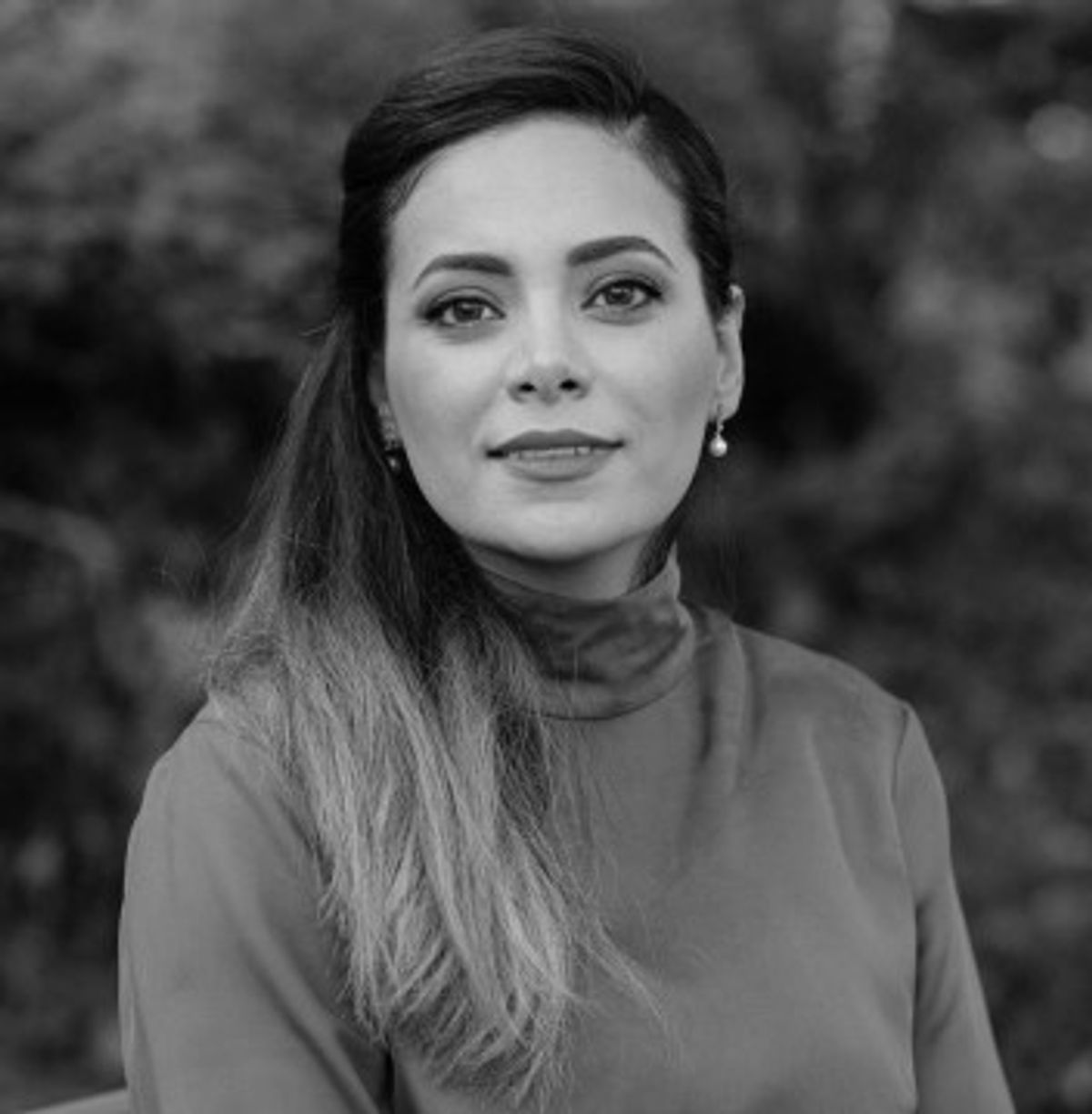
Inferring cell health from structure and morphology is second nature for Maddison Masaeli, an engineer scientist and chief executive officer at Deepcell. “The way that cells look has been integral to biology since the discovery of cells,” she said. “It goes all the way from getting a sense about how cells are doing in a culture—whether they're healthy and living and thriving—all the way to diagnosing and staging cancer in a pathology or cytology setting.”
When Masaeli worked as a postdoctoral researcher for Euan Ashley, a cardiovascular expert at Stanford University, she studied cardiomyopathy models. Her work relied heavily on phenotypic analysis to determine cardiomyocyte maturity and function. “The tools that we had available as scientists were extremely limited, even to the degree that we couldn't even measure a basic volume of cells,” she said.
She sought to leverage computer vision and deep learning to help tackle those challenges, and after seeing their success, Masaeli cofounded Deepcell in 2017. She and her team developed an AI-based image analysis platform trained on large datasets of about two billion image data points gathered from cells originating from different tissues from both healthy people and patients with diseases.
According to Masaeli, their disease agnostic platform can detect abnormalities in the morphology of any cell type, which enables a wide range of applications in research and medicine. Some diseases have an obvious connection to cell morphology (for example, tumor cells structurally differ from healthy cells), but finding unexpected connections in other diseases excites Masaeli. For example, in one customer study on aging, the model picked up morphological differences between cells from old patients and those from young patients. After exposing the old-patient cells to drugs being tested to revert aging, Masaeli noted that the treated old-patient cells resembled the morphology of young-patient cells.
“This is just fascinating [to find] the most non-obvious applications that could be very minute changes in morphology that we didn't have tools to evaluate directly,” said Masaeli.
Predictive AI in precision medicine
While AI use cases have sprouted across diverse basic research areas, from single cell studies to neural network models that decode language, most researchers have their eyes on the prize: improving human health.
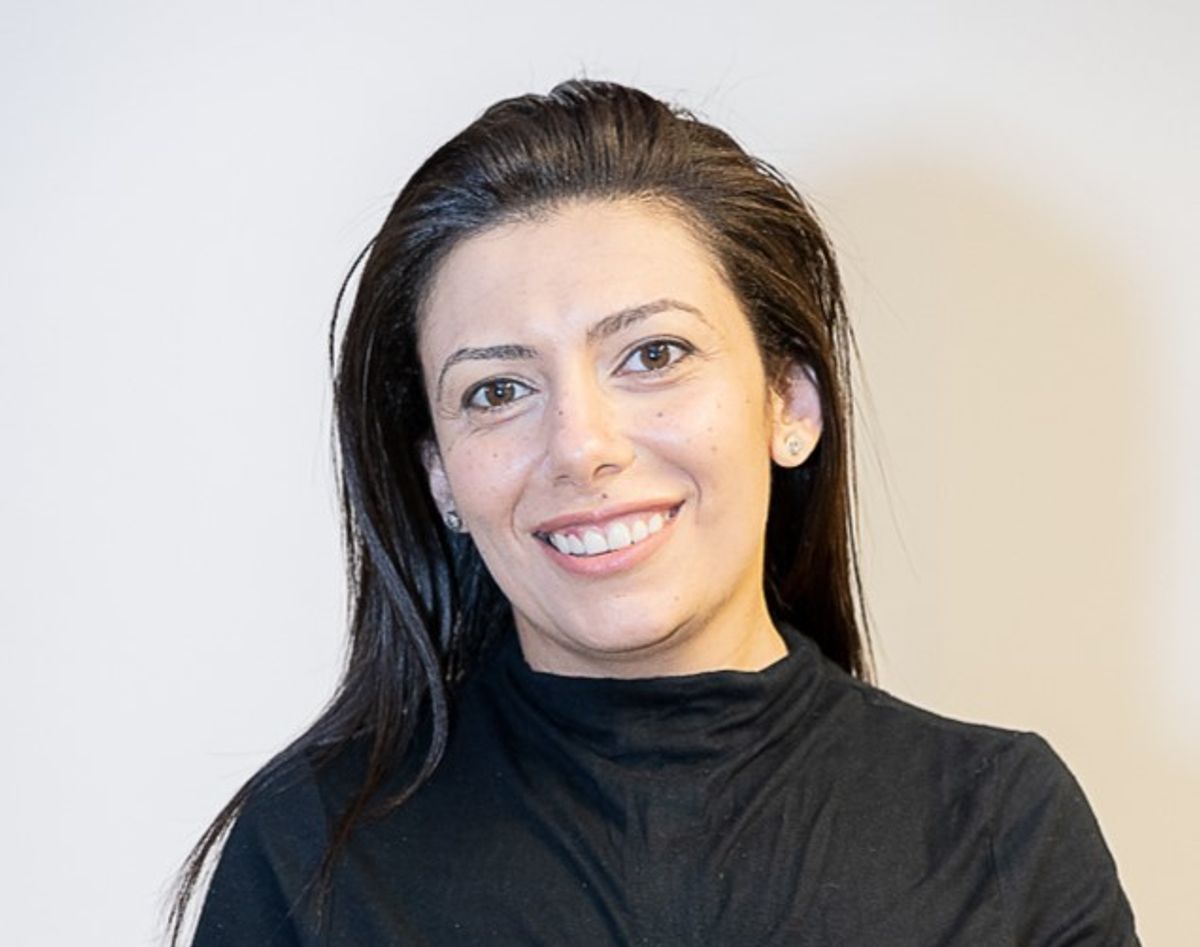
Nardin Nakhla, a neuroscientist and chief technology officer at Simmunome, intends to fix the leaky drug discovery pipeline. “In the pharma industry, 90 percent of drugs fail, and only 10 percent make it all the way to the market. There's a lot of trial and error,” said Nakhla.
A lot of work goes into drug screening and determining the right drug, but sometimes a drug doesn’t work because the developers picked the wrong target or causal pathway. Nakhla and her team focus on the early stages of the workflow to minimize downstream losses. They trained their models on how biology works at the molecular level so that the models better understand pathways and can identify causal targets. The team can then simulate the downstream influence of a drug on a pathway and estimate its efficacy in stopping disease progression. “The idea is to provide this tool, so instead of [drug developers] trying five times before they get it right, maybe we can get it right from the first or second time,” said Nakhla.
In preliminary tests, the team compared the efficacies of drugs tested in 24 oncology clinical trials with prediction data from their simulations. They found that their models predicted drug efficacies with almost 70 percent accuracy. The Simmunome team intends to conduct more tests in the near future to ensure robust predictions in other disease areas.
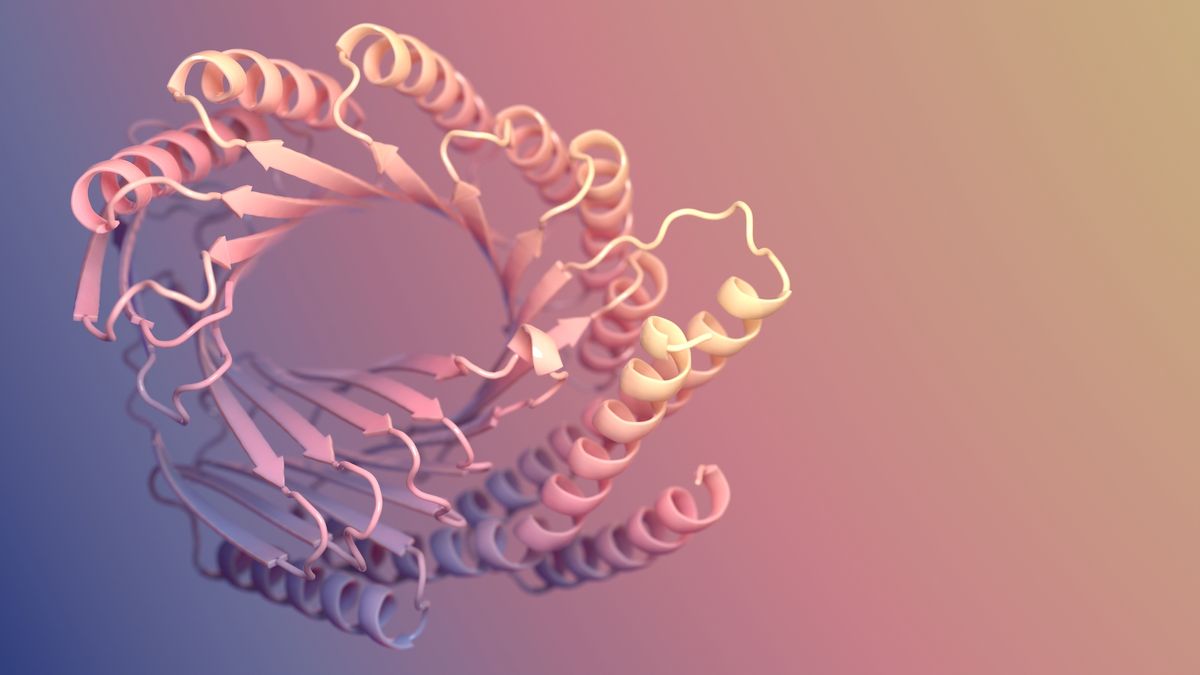
While Nakhla hopes to streamline conventional drug discovery processes, Ideker envisions a new world in medicine that includes customized patient therapies. A patient with breast cancer, for instance, may possess up to 50 genetic mutations that alter her response to standard medications. Given that genomic signatures differ between patients, researchers and physicians need the right combination of AI models and genomic data to appropriately treat such a complex perturbation of the system, according to Ideker. His team develops algorithms that could analyze a patient’s genomic mutations to inform the right treatment course.10
“Essentially, what it's doing is determining or making a prediction on which drugs will produce a response to that patient, and which drugs are likely to not produce a response,” said Ideker. In the future, as researchers build more sophisticated AI models, Ideker believes that there will be an armada of clinical trials where patients could avail themselves of personalized medicines catered to their genomes, maximizing the treatment response. “Why is it that Netflix is able to give you recommendations for what movies you're going to like to watch tonight, but your clinician can't get you AI guided recommendations for therapies for how you should be treated?” questioned Ideker.
AI advances: proceed with caution
Today, there is no dearth of appreciation for AI in biology from researchers, investors, and the public. That was not always the case. Ideker recalled that being an early bird in this field was frustrating due to the uphill climb of peer acceptance. “If you've correctly identified what the gap is, and you are trying to push the field forward, there's always resistance,” he said. “It’s been hard, but it should be.”
Although Ideker is happy that biologists are finally warming up to AI, he thinks that some may have veered too far. The hype has gotten to a point where researchers cannot start a new venture without mentioning AI, he joked.
“Everybody thinks that now they need to solve their problem one way or another with AI. And sometimes those problems might not be a great fit for AI and deep learning,” agreed Masaeli, who experienced a similar skepticism-to-optimism journey. According to her, there is a lot that AI could help achieve in certain topics, but she urged researchers working in areas where large datasets aren’t available to evaluate existing tools rather than forcing AI-based approaches.
Whether researchers use AI methodologies or any other techniques, they need to possess a deep understanding of their topic to succeed, according to Baker. “People were surprised that we transitioned so quickly from physically based models to deep learning models,” he said. This was only possible because the researchers had worked on protein design for several years, understood the limitations and possibilities that came with the territory, and developed an intuition for the system, he explained. “If you understand the scientific problem, then AI is just another tool.”
- Senior AW, et al. Improved protein structure prediction using potentials from deep learning. Nature. 2020;577:706–710.
- Jumper J, et al. Highly accurate protein structure prediction with AlphaFold. Nature. 2021;596:583–589.
- Baek M, et al. Accurate prediction of protein structures and interactions using a three-track neural network. Science. 2021;373(6557):871-876.
- Kuhlman B, et al. Design of a novel globular protein fold with atomic-level accuracy. Science. 2003;302(5649):1364-1368.
- Huang PS, et al. The coming of age of de novo protein design. Nature. 2016;537(7620):320-327.
- Ferruz N, et al. ProtGPT2 is a deep unsupervised language model for protein design. Nat Commun. 2022;13(1):4348.
- Watson JL, et al. De novo design of protein structure and function with RFdiffusion. Nature. 2023;620:1089–1100.
- Madani A, et al. Large language models generate functional protein sequences across diverse families. Nat Biotechnol. 2023;41(8):1099-1106.
- Ueda G, et al. Tailored design of protein nanoparticle scaffolds for multivalent presentation of viral glycoprotein antigens. Elife. 2020;9:e57659.
- Zhao X, et al. Cancer mutations converge on a collection of protein assemblies to predict resistance to replication stress. Cancer Discov. 2024.